In discussions of new technological advances that can no doubt aid businesses and organisations in their journey to success, risks undeniably come in.
With every change comes uncertainty, and with such comes talks on how to manage and mitigate risks to be able to emerge from challenges as unscathed as possible.
One good thing to do in times of changes is to see such shifts as opportunities rather than just mere troubles to surpass for the business.
Generative artificial intelligence has created buzz in the past few months and it has made its way to the Finance function, imposing possible hurdles and challenges along the way which chief financial officers and leaders must definitely look into.
In this regard, such risks should also be looked upon as chances for improvement.
Gerry Chng, risk advisory executive director at Deloitte Singapore, sees that one of the key uses of Generative AI is the ability to create synthetic data to augment existing data points in a more cost-effective manner, which in turn makes it easier to conduct financial modelling and risk analysis.
Financial modelling and risk analysis
Chng says financial modelling and risk analysis typically rely on the ability to generate massive data points to computationally evaluate different scenarios and its potential impact on the outcomes.
"The creation of such data points can be a laborious process if firms do not have sufficient data points to develop a robust model for simulations."
For this reason, Chng thinks traditional machine learning algorithms have been used to generate synthetic data based on creating samples that are representative of the actual data. However, he points out that they are limited to structured data points that are observable within the existing datasets.
In addition, risk management processes typically deal a lot with information processing and preparation, which Chng says makes everything time-consuming for analysts to sift through and extract thematic issues that may not be immediately obvious.
"Generative AI technology allows solutions to be built that can process massive volumes of information such as news articles, annual reports, and analyst forecasts, making it easier for analysts to uncover trends and topical grouping of information," he says.
While Generative AI does not replace the role of analysts, Chng notes that it augments their ability to make sense of such textual, audio, and video information. Depending on the results presented, the analyst can then zoom in on topics of interest to better understand the situation and any significant risks that they may represent.
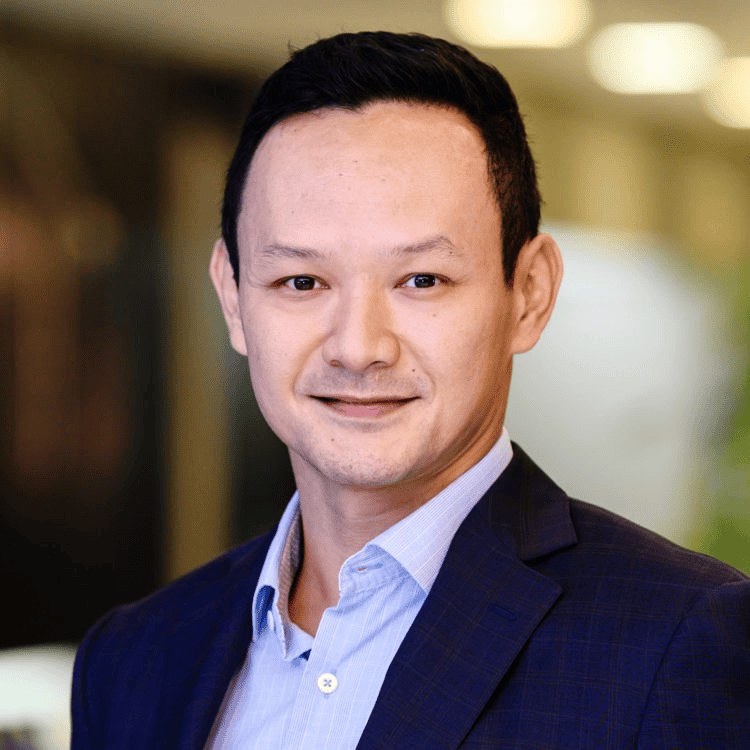
Risks and GenAI
"The risks of Generative AI, and more generally, the use of AI are well known and have taken on more prominence in the past year, with some of the emerging regulations around the world such as the EU AI Act, and the more recent reversal in position from the UK Government on regulating AI centres around the use of "General Purpose" or "Frontier" models comprising of such Large Language Models," Chng explains.
He adds that some of the significant risks revolve around unanticipated biases in the outputs as there is currently a lack of transparency in the data that had been used in the training and any subsequent alignment that had been performed on the models before they were published for consumer usage.
Chng further explains that there are also inherent risks of inaccuracies from the models due to their statistical nature, which is more a feature of such technology rather than a bug.
A human-centric approach
Understanding the limitations of AI is important so that firms can do the proper planning to use such technology as part of their business operations.
Chng believes a human-centric approach that focuses on the following is a good starting point to ensure that the technology is able to add value while managing the risks involved:
- Ensure there is sufficient awareness and training across the enterprise in the use of such technology, which has differences from "traditional software" that may not be immediately obvious to users.
- Understand the impact of inaccuracies of the output to the business.
- In designing applications leveraging Generative AI, ensuring the use of good human-centred user experience (UX) design helps users understand that while the outcomes produced may be probabilistically correct, they are
andare not necessarily factual. This should factor into user onboarding, processing, and feedback mechanisms to allow users to make future corrections when the model's output is not what is expected. There is some good research by technology firms such as Google's People + AI Research that covers such design principles.
Finance and GenAI
Chng says one of the popular applications of Generative AI leverages the ability to connect to a firm's internal data stores. This opens up opportunities to augment internal teams when there is a need to reference such information.
He gives call centre operations as an example wherein they can leverage Generative AI across the process lifecycle to enhance the experience and process in various ways such as speech-to-text algorithms, sentiment analysis, and coaching mechanisms.
Further, Chng says Generative AI has seen pilot adoption in helping development teams code more consistently and efficiently through the concept of co-pilots that can help in creating portions of code as well as creating technical documentation based on the code that has been developed. This enhances the ability of an existing team to develop competitive solutions with a shorter time-to-market.