When it comes to AI in corporate finance, there are five top use cases for FP&A leaders to consider, said Gartner recently.
According to the research firm, the top five use cases include demand/revenue forecasting, anomaly and error detection, decision support POC (percentage of completion) revenue forecasting, and cash collections.
Organisations ignoring these use cases should have a good reason for doing so because they offer the best combination of feasibility and business benefit, said Mark D. McDonald, senior director, research in the Gartner Finance practice.
“Looking to apply AI to other use cases before getting these five working effectively is likely leaving process efficiency and business performance gains on the table,”he noted.
According to Gartner, its analysts examined 23 uses cases related to AI in corporate finance that represents the types of processes a future-looking autonomous finance organisation will work on.
The use cases were ranked according to their business value and feasibility of implementation, the advisory firm said.
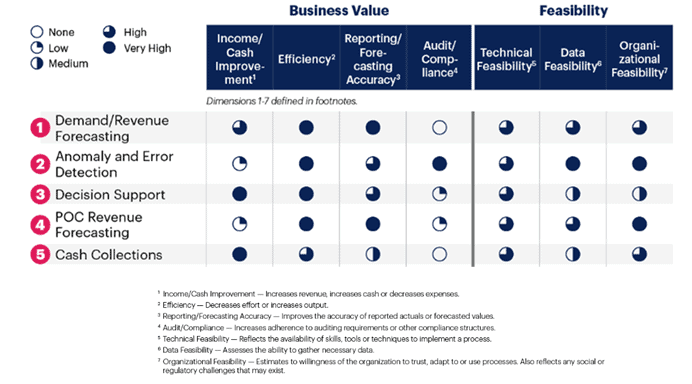
“FP&A Leaders should take into account the maturity and needs of their own finance organisation because the applicability may vary across organizations and industries,” McDonald advised.
These use cases for AI in corporate finance are commonly implemented and effective, but the most valuable use cases exploit a company's unique strengths and allow it to further differentiate itself, he added.
To clarify the use cases, Gartner provided more detailed definitions as follows.
Demand / revenue forecasting. Using both internal and external sources of data, models predict demand and associated revenue across a variety of dimensions including business unit, product line, SKU, customer type and region.
Anomaly and error detection. Anomaly detection uses a series of machine learning (ML) models to highlight transactions or balances that are in error or potentially violate accounting principles or policies. A comprehensive solution will also include real-time analysis during data entry preventing errors from entering the workflow and avoiding costly downstream corrections.
Decision support. ML prediction algorithms designed to predict outcomes based on current data are used to predict outcomes when alternative data values are used. Using models with hypothetical data predicts the result of alternate decisions.
POC revenue forecasting or POC accounting. ML models forecast the percentage-of-completion metrics (for instance, hours, cost, units, and weight) to predict POC revenue and the total completion effort remaining.
Cash collections. ML models are used to forecast when customers will pay invoices triggering proactive collection efforts before payments are past due. Using the predictions from these models, collections staff focus their efforts on at-risk accounts. Forecast cash collections also contribute to overall ML-driven cashflow forecasting.
Forecasting is a popular use case in finance departments because legacy processes are manually intensive and notoriously unreliable and AI excels at automation and improving accuracy, McDonald said.
"Many pre-configured software packages address common finance processes such as accounts receivable and accounts payable but be aware that use cases which address unique business needs, such as forecasting, will require some internal skills to build,” he advised.