At the launch of a recent report examining the effectiveness of financial fraud risk management, Julie Bell Lindsay - the Executive Director at the Center for Audit Quality — was quoted as saying that “the risk of financial statement fraud at public companies is real, and that risk has only increased during the pandemic.”
The risks associated with financial fraud that modern CFOs face today is particularly high. The same report examined Accounting and Auditing Enforcement Releases (AAERs) issued by the Securities and Exchange Commission (SEC) in the US from 2014 to 2019 and found that while the SEC frequently charged the person directly responsible for perpetuating the financial fraud, the CFO was among the most commonly charged employees.
Data analytics techniques can play an important role in mitigating the risk of financial fraud for CFOs.
The Committee of Sponsoring Organizations of the Treadway Commission (COSO) fraud risk management guide explicitly acknowledges the value that data analytics can bring in a fraud risk management program.
In particular, it highlights that data analytics can support fraud risk assessments by identifying red flags or potential high-risk areas, validating the correct identification of a scheme or the validity of risk assessment process findings, and being used to develop techniques to monitor high-risk or improper behaviors.
Given the rapid advancement in technology and the growth in both the quantity and variety of data available in the corporate setting, the use of data analytics techniques have become widespread in accounting, including in the area of fraud detection. In this article, we examine two techniques - cluster analysis and Benford’s law based tests - which can play a role in financial fraud detection.
Cluster Analysis
One particularly important data analytics technique in fraud detection is the use of cluster analysis to detect anomalies in financial data.
Anomalies in data refer to outlier observations that deviate so much from other observations that they may have been generated by a different mechanism from the bulk of the other data. Anomaly detection techniques are effective in fraud detection because they can easily identify unusual corporate financial behaviour.
Cluster analysis involves classifying data in such a way that data assigned to the same cluster (or group) are more similar to one another (along dimensions being examined) than to data assigned to another cluster.
In cluster analysis, anomalous data items are assigned to clusters which are small and/or sparse while normal data items are assigned to clusters which are large and dense.
Accordingly, investors focus much of their attention on identifying clusters which are small and/or sparse when attempting to detect financial fraud.
To illustrate how cluster analysis can be used as part of a fraud detection programme, we performed the analysis on a sample of claims data.
When examining claims data for potential fraud, investigators often seek to corroborate that the time period between the date indicated on a receipt and the date in which the corresponding claim was submitted is not be overly long, consistent with claims being submitted promptly after the corresponding expenses have been incurred.
The time period between the date that a claim was submitted and the date on which a reimbursement was made should also not be overly short or long, and should instead be consistent with a reasonable processing period. The claim amount should also not be overly large or small.
Figure 1 presents the results of the cluster analysis performed on three pertinent dimensions of the data: (i) the claim amount (CLM_AMT), (ii) the number of days between the date indicated on a receipt submitted with a claim and date that the claim was submitted (DAYSTO_CLAIM), and (iii) the number of days between the date that a claim was submitted and the date on which a reimbursement was made (DAYSTO_REIMBURSE).
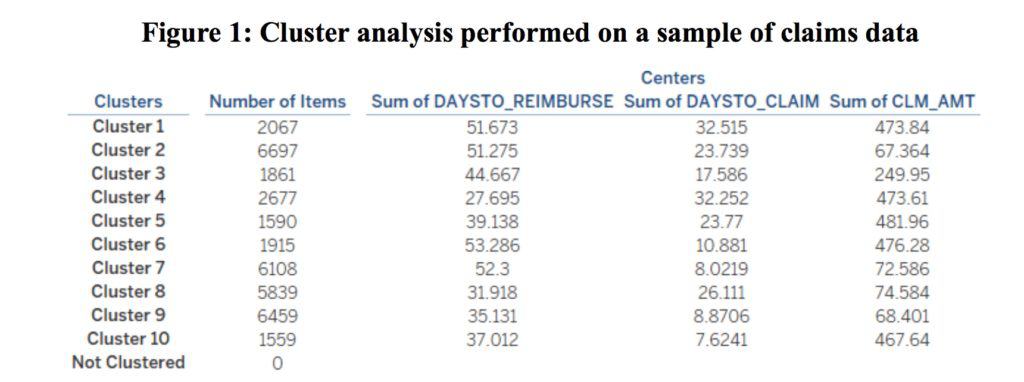
In examining Figure 1, we identify cluster 10 as potentially containing anomalous data items. Specifically, Cluster 10 is the smallest cluster formed by the cluster analysis, with only 1559 data items assigned to the cluster. These 1599 data items account for only 4.23% of data items in our dataset.
In comparison, the 6697 data items in the largest cluster (cluster 2) account for 18.22% of data items in our dataset. We also find that the Cluster 10 has a relatively low DAYSTO_REIMBURSE of 37.01 days (this is the fourth lowest among the 10 clusters), a relatively low DAYSTO_CLAIM of 7.62 days (this is the lowest among the 10 clusters), and a relatively high CLM_AMT of $467.64 (this is the fourth highest among the 10 clusters).
With these insights, a fraud investigator could conduct further analysis into the transactions in cluster 10 to determine if fraud has been perpetuated.
Benford’s Law
Benford’s law based tests are another important data analytics technique that can be employed in forensic accounting to detect anomalies in data. Benford’s Law provides that in a randomly generated set of data, numbers within the dataset should have 1 as the most frequently appearing first digit (about 30.1% of the time) and have 9 as the least frequently appearing first digit (about 4.6% of the time).
Knowledge of Benford’s law allows forensic investigators to design related tests that can effectively detect anomalies in data.
Specifically, Benford’s law based tests represent tests of abnormal duplication, where the actual frequencies with which digits appear in numbers in a dataset are tabulated and compared with the expected distribution of these digits as predicted by Benford’s law.
Where significant deviations between these actual and expected frequencies are detected, Benford’s law tests would highlight these deviations as anomalies that should be investigated for potential fraud.
To illustrate how Benford’s law can be used as part of a fraud detection programme, we performed Benford’s law on a set of sales transaction data. Figure 2 presents the results of the analysis. The blue bars represent the percentage of sales transactions with first digits which correspond to integers from 1 to 9.
The orange line represents the percentage of sales transactions that are predicted to correspond to integers from 1 to 9 by Benford’s law. Inspecting the visualisation presented in Figure 2 will allow a forensic investigator to easily detect deviations from Benford’s Law in the data.
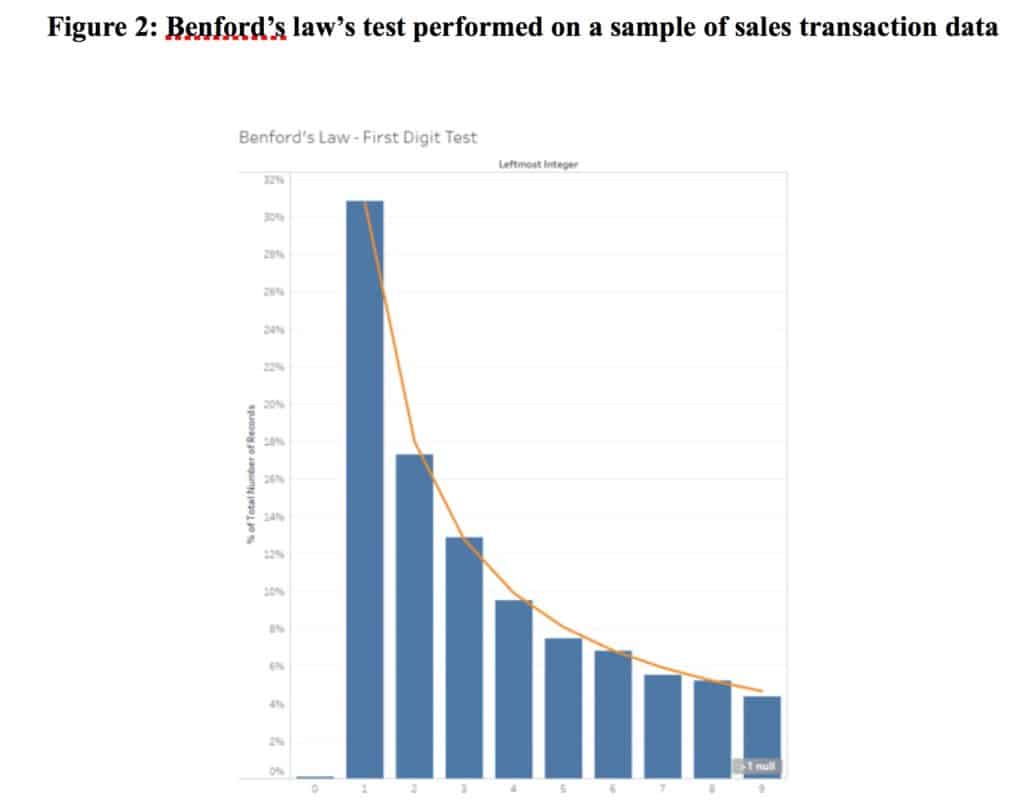
The way forward with data analytics
Financial fraud is a key risk that CFOs have to confront. While fraudsters often go to great lengths to hide their tracks and prevent detection, data analytics represents a key tool which CFOs can use to mitigate financial fraud risk.
Given improvements in technology and the growing prevalence of data availability, fraud investigators can now effectively apply data analytics techniques – such as cluster analysis and Benford’s law based tests - to detect anomalies in complex datasets.
Often, these data analytics techniques are not used in isolation, but are instead used in combination with other techniques. While anomaly detection techniques can be useful in fraud investigations because they highlight unusual corporate financial behaviour, the fraud investigator should note that not all anomalous data represent fraud – there may be legitimate reasons that account for these data items’ characteristics and investigators should further investigate these data items using complementary forensic analysis techniques to ascertain the nature of the underlying data.