Finance leaders must temper their expectations around generative artificial intelligence (GenAI) even amid the significant hype in the market, according to Gartner.
As CFOs look to maximise resources and improve efficiency and decision making through the said technological advancement, Gartner says this can likely lead to a period of disillusionment with various technologies in this space.
Although Gartner experts forecast disillusionment with GenAI tools in finance in the future, this isn’t to say the technology won’t be useful in finance.
On the contrary, GenAI has several applications for finance professionals, they just might not be as transformative as many finance leaders think right now. GenAI uses text as its source, so for tasks that require analysis of text, such as contract analysis, it excels.
The Gartner Hype Cycle for Finance AI and Advanced Analytics showcases the leading innovations revolutionising finance.
This Hype Cycle provides CFOs with the current state of key AI and advanced analytics techniques relevant to finance, helping them align technology roadmaps with business strategy. While many of the innovations featured are readily available and widely used today, others are forward-looking and present the greatest promise for the future.
According to Gartner, CFOs can use this Hype Cycle to build a finance transformation roadmap that delivers short-term value while simultaneously preparing for the future.
"At the very Peak of Inflated Expectations in finance is generative AI," says Mark D. McDonald, senior director analyst in the Gartner finance practice.
"A range of publicly available generative AI (GenAI) tools have generated enormous publicity for the technology in the last two years, but as finance functions adopt this technology, they may not find it as transformative as expected."
Figure 1: Hype Cycle for Finance AI and Advanced Analytics, 2024
![[Image Alt Text for SEO]](https://emt.gartnerweb.com/ngw/globalassets/en/newsroom/images/graphs/hcfinai24.png)
Source: Gartner (August 2024)
McDonald says finance functions could also use GenAI to do things they currently don’t, citing the comparison of an inbound vendor invoice with the negotiated pricing to ensure charges align with the agreed prices.
"The main strengths of GenAI in finance are its ease of access and simplicity of use. With many vendors offering private in-house GenAI solutions, harnessing such tools is largely a case of teaching employees how to use it and under what circumstances it is a reliable solution."
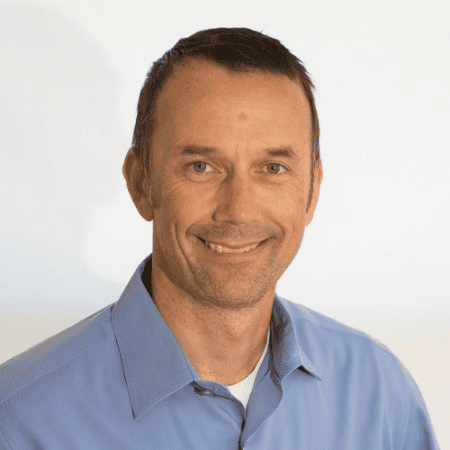
When it comes to tasks that are based in numerical data, however, finance functions will need to rely on other AI techniques: most notably various applications of machine learning.
Machine learning can help finance professionals with tasks like forecasting revenue or finding errors in large volumes of data.
McDonald notes that this can also help with new more sophisticated methods of analyzing our financial results, detecting trends that otherwise could be missed.
"One of the main benefits of machine learning is that finance leaders can quantify the quality of the algorithm's output which can serve as evidence for auditable transactions."
Using machine learning will require some new skills, however. Finance organisations are beginning to employ the citizen data science model that teaches finance professionals a subset of the data science capability and the skills to employ fundamental data science techniques.
Composite AI
Composite AI is at the Innovation Trigger stage of the Hype Cycle, and Gartner refers to it as the combined application of different AI techniques to improve the efficiency of learning to broaden the level of knowledge representations.Â
"As AI adoption matures in finance functions, it will become clear that no single AI technique is a panacea," says McDonald. "Combining AI techniques is much more effective than relying only on heuristics or a fully data-driven approach."
The growing reliance on AI for decision making is driving organisations toward composite AI because the most appropriate actions can be better determined by combining rule-based and optimisation models — a combination often referred to as prescriptive analytics. Small datasets, or the limited availability of data, have also pushed organizations to combine multiple AI techniques.